Independet Component Analysis with Nilearn (healthy subjects)¶
from nilearn.decomposition import CanICA
from nilearn.plotting import plot_prob_atlas
from nilearn.image import iter_img
from nilearn.plotting import plot_stat_map, show, plot_prob_atlas, plot_matrix, plot_connectome, find_probabilistic_atlas_cut_coords
/home/jt/.local/lib/python3.8/site-packages/nilearn/datasets/__init__.py:86: FutureWarning: Fetchers from the nilearn.datasets module will be updated in version 0.9 to return python strings instead of bytes and Pandas dataframes instead of Numpy arrays.
warn("Fetchers from the nilearn.datasets module will be "
Data import¶
We are working with 2 different datasets, so we created a list with the corresponding files. We exluded 3 subjects, subject 17, 39 and 48 because of to much movement or artefacts.
files_list=[]
all_list=list(range(1,73))
healthy_list=list(range(52,73))
dep_list=list(range(1,52))
exclution_list=[17,39,48]
for i in healthy_list:
if i not in exclution_list:
j="{:02d}".format(i)
files_list.append("/media/jt/Daten1/preproc/datasink/smooth/sub-"+j+"/task-restingstatewithclosedeyes/fwhm-8_swarsub-"+j+"_task-rest_bold.nii")
Checking the subject list if everything worked well. In this case we are working with the depressiv group.
for i in files_list:
print (i)
/media/jt/Daten1/preproc/datasink/smooth/sub-52/task-restingstatewithclosedeyes/fwhm-8_swarsub-52_task-rest_bold.nii
/media/jt/Daten1/preproc/datasink/smooth/sub-53/task-restingstatewithclosedeyes/fwhm-8_swarsub-53_task-rest_bold.nii
/media/jt/Daten1/preproc/datasink/smooth/sub-54/task-restingstatewithclosedeyes/fwhm-8_swarsub-54_task-rest_bold.nii
/media/jt/Daten1/preproc/datasink/smooth/sub-55/task-restingstatewithclosedeyes/fwhm-8_swarsub-55_task-rest_bold.nii
/media/jt/Daten1/preproc/datasink/smooth/sub-56/task-restingstatewithclosedeyes/fwhm-8_swarsub-56_task-rest_bold.nii
/media/jt/Daten1/preproc/datasink/smooth/sub-57/task-restingstatewithclosedeyes/fwhm-8_swarsub-57_task-rest_bold.nii
/media/jt/Daten1/preproc/datasink/smooth/sub-58/task-restingstatewithclosedeyes/fwhm-8_swarsub-58_task-rest_bold.nii
/media/jt/Daten1/preproc/datasink/smooth/sub-59/task-restingstatewithclosedeyes/fwhm-8_swarsub-59_task-rest_bold.nii
/media/jt/Daten1/preproc/datasink/smooth/sub-60/task-restingstatewithclosedeyes/fwhm-8_swarsub-60_task-rest_bold.nii
/media/jt/Daten1/preproc/datasink/smooth/sub-61/task-restingstatewithclosedeyes/fwhm-8_swarsub-61_task-rest_bold.nii
/media/jt/Daten1/preproc/datasink/smooth/sub-62/task-restingstatewithclosedeyes/fwhm-8_swarsub-62_task-rest_bold.nii
/media/jt/Daten1/preproc/datasink/smooth/sub-63/task-restingstatewithclosedeyes/fwhm-8_swarsub-63_task-rest_bold.nii
/media/jt/Daten1/preproc/datasink/smooth/sub-64/task-restingstatewithclosedeyes/fwhm-8_swarsub-64_task-rest_bold.nii
/media/jt/Daten1/preproc/datasink/smooth/sub-65/task-restingstatewithclosedeyes/fwhm-8_swarsub-65_task-rest_bold.nii
/media/jt/Daten1/preproc/datasink/smooth/sub-66/task-restingstatewithclosedeyes/fwhm-8_swarsub-66_task-rest_bold.nii
/media/jt/Daten1/preproc/datasink/smooth/sub-67/task-restingstatewithclosedeyes/fwhm-8_swarsub-67_task-rest_bold.nii
/media/jt/Daten1/preproc/datasink/smooth/sub-68/task-restingstatewithclosedeyes/fwhm-8_swarsub-68_task-rest_bold.nii
/media/jt/Daten1/preproc/datasink/smooth/sub-69/task-restingstatewithclosedeyes/fwhm-8_swarsub-69_task-rest_bold.nii
/media/jt/Daten1/preproc/datasink/smooth/sub-70/task-restingstatewithclosedeyes/fwhm-8_swarsub-70_task-rest_bold.nii
/media/jt/Daten1/preproc/datasink/smooth/sub-71/task-restingstatewithclosedeyes/fwhm-8_swarsub-71_task-rest_bold.nii
/media/jt/Daten1/preproc/datasink/smooth/sub-72/task-restingstatewithclosedeyes/fwhm-8_swarsub-72_task-rest_bold.nii
Run ICA¶
Now we are running the ICA (indipendent component analysis with 20 different components. We made this twice, once with the depressiv group and once with the healthy control group.
canica = CanICA(n_components=20,
memory="/media/jt/Daten1/preproc/ICA_nilearn/nilearn_cache", memory_level=2,
verbose=10,
smoothing_fwhm=8,
n_jobs=-2,
mask_strategy='template',
random_state=0)
canica.fit(files_list)
# Retrieve the independent components in brain space. Directly
# accesible through attribute `components_img_`.
canica_components_img = canica.components_img_
# components_img is a Nifti Image object, and can be saved to a file with
# the following line:
canica_components_img.to_filename('//media/jt/Daten1/preproc/ICA_nilearn/canica_resting_state_healthy.nii.gz')
[MultiNiftiMasker.fit] Loading data from [/media/jt/Daten1/preproc/datasink/smooth/sub-52/task-restingstatewithclosedeyes/fwhm-8_swarsub-52_task-rest_bold.nii,
/media/jt/Daten1/preproc/datasink/smooth/sub-53/task-restingstatewithclosedeyes/fwhm-8_swarsub-53_task-rest_bold.nii,
/media/jt/Daten1/preproc/datasink/smooth/sub-54/task-restingstatewithclosedeyes/fwhm-8_swarsub-54_task-rest_bold.nii,
/media/jt/Daten1/preproc/datasink/smooth/sub-55/task-restingstatewithclosedeyes/fwhm-8_swarsub-55_task-rest_bold.nii,
/media/jt/Daten1/preproc/datasink/smooth/sub-56/task-restingstatewithclosedeyes/fwhm-8_swarsub-56_task-rest_bold.nii,
/media/jt/Daten1/preproc/datasink/smooth/sub-57/task-restingstatewithclosedeyes/fwhm-8_swarsub-57_task-rest_bold.nii,
/media/jt/Daten1/preproc/datasink/smooth/sub-58/task-restingstatewithclosedeyes/fwhm-8_swarsub-58_task-rest_bold.nii,
/media/jt/Daten1/preproc/datasink/smooth/sub-59/task-restingstatewithclosedeyes/fwhm-8_swarsub-59_task-rest_bold.nii,
/media/jt/Daten1/preproc/datasink/smooth/sub-60/task-restingstatewithclosedeyes/fwhm-8_swarsub-60_task-rest_bold.nii,
/media/jt/Daten1/preproc/datasink/smooth/sub-61/task-restingstatewithclosedeyes/fwhm-8_swarsub-61_task-rest_bold.nii,
/media/jt/Daten1/preproc/datasink/smooth/sub-62/task-restingstatewithclosedeyes/fwhm-8_swarsub-62_task-rest_bold.nii,
/media/jt/Daten1/preproc/datasink/smooth/sub-63/task-restingstatewithclosedeyes/fwhm-8_swarsub-63_task-rest_bold.nii,
/media/jt/Daten1/preproc/datasink/smooth/sub-64/task-restingstatewithclosedeyes/fwhm-8_swarsub-64_task-rest_bold.nii,
/media/jt/Daten1/preproc/datasink/smooth/sub-65/task-restingstatewithclosedeyes/fwhm-8_swarsub-65_task-rest_bold.nii,
/media/jt/Daten1/preproc/datasink/smooth/sub-66/task-restingstatewithclosedeyes/fwhm-8_swarsub-66_task-rest_bold.nii,
/media/jt/Daten1/preproc/datasink/smooth/sub-67/task-restingstatewithclosedeyes/fwhm-8_swarsub-67_task-rest_bold.nii,
/media/jt/Daten1/preproc/datasink/smooth/sub-68/task-restingstatewithclosedeyes/fwhm-8_swarsub-68_task-rest_bold.nii,
/media/jt/Daten1/preproc/datasink/smooth/sub-69/task-restingstatewithclosedeyes/fwhm-8_swarsub-69_task-rest_bold.nii,
/media/jt/Daten1/preproc/datasink/smooth/sub-70/task-restingstatewithclosedeyes/fwhm-8_swarsub-70_task-rest_bold.nii,
/media/jt/Daten1/preproc/datasink/smooth/sub-71/task-restingstatewithclosedeyes/fwhm-8_swarsub-71_task-rest_bold.nii,
/media/jt/Daten1/preproc/datasink/smooth/sub-72/task-restingstatewithclosedeyes/fwhm-8_swarsub-72_task-rest_bold.nii]
[MultiNiftiMasker.fit] Computing mask
[MultiNiftiMasker.transform] Resampling mask
[CanICA] Loading data
[Memory]1.1s, 0.0min : Loading randomized_svd from /media/jt/Daten1/preproc/ICA_nilearn/nilearn_cache/joblib/sklearn/utils/extmath/randomized_svd/0f87f7ff1f1b2f75bba094491bd37bc5
______________________________________randomized_svd cache loaded - 0.1s, 0.0min
[Parallel(n_jobs=-2)]: Using backend LokyBackend with 7 concurrent workers.
[Parallel(n_jobs=-2)]: Done 3 out of 10 | elapsed: 1.4s remaining: 3.2s
[Parallel(n_jobs=-2)]: Done 5 out of 10 | elapsed: 1.5s remaining: 1.5s
[Parallel(n_jobs=-2)]: Done 7 out of 10 | elapsed: 1.7s remaining: 0.7s
[Parallel(n_jobs=-2)]: Done 10 out of 10 | elapsed: 1.9s finished
/home/jt/.local/lib/python3.8/site-packages/nilearn/image/image.py:1054: FutureWarning: The parameter "sessions" will be removed in 0.9.0 release of Nilearn. Please use the parameter "runs" instead.
data = signal.clean(
In case you don’t make this whole script in one time you can start here now loading the created ICA file to continue.
# Only run for plotting without processing or further processing of the ICA data without CanICA them again
canica_components_img="/media/jt/Daten1/preproc/ICA_nilearn/canica_resting_state_healthy.nii.gz"
ICA plotting¶
Here we plot the calculated components by CanICA.
# Plot all ICA components together
plot_prob_atlas(canica_components_img, title='All ICA components')
/usr/local/lib/python3.8/dist-packages/numpy/ma/core.py:2825: UserWarning: Warning: converting a masked element to nan.
_data = np.array(data, dtype=dtype, copy=copy,
/home/jt/.local/lib/python3.8/site-packages/nilearn/plotting/displays.py:101: UserWarning: linewidths is ignored by contourf
im = getattr(ax, type)(data_2d.copy(),
/home/jt/.local/lib/python3.8/site-packages/nilearn/plotting/displays.py:101: UserWarning: No contour levels were found within the data range.
im = getattr(ax, type)(data_2d.copy(),
<nilearn.plotting.displays.OrthoSlicer at 0x7fc04b6cb0d0>
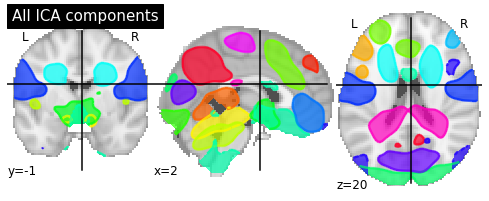
#plot the different slices of components
for i, cur_img in enumerate(iter_img(canica_components_img)):
plot_stat_map(cur_img, display_mode="z", title="IC %d" % i,
cut_coords=1, colorbar=False)
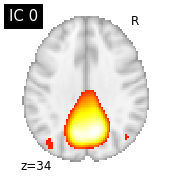
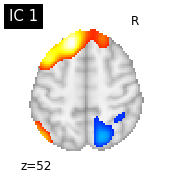
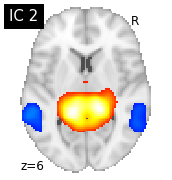
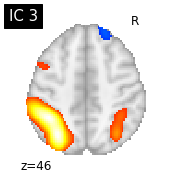
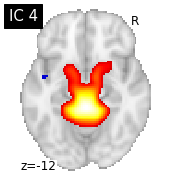
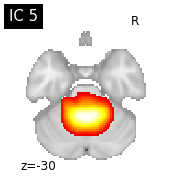
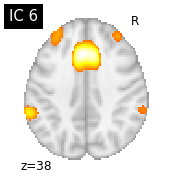
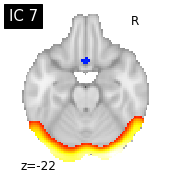
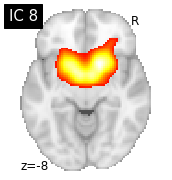
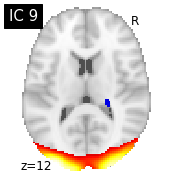
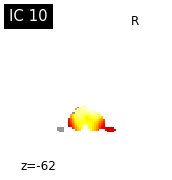
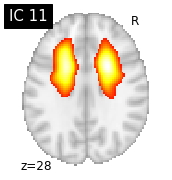
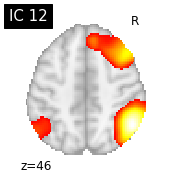
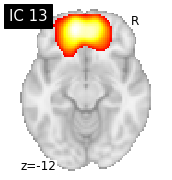
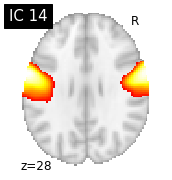
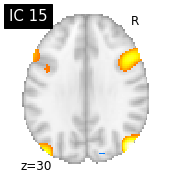
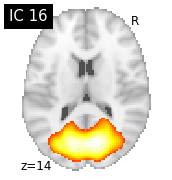
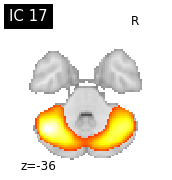
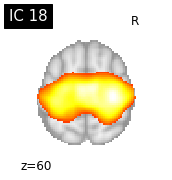
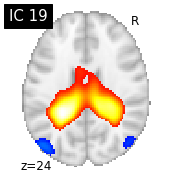
Region extraction¶
Next step is to extract the different regions of the 20 components. Therefore we used the nilearn region extractor with a min region size of 2000. That corresponds to about 100 voxel (2x2x5mm voxel size).
components_img=canica_components_img
# Import Region Extractor algorithm from regions module
# threshold=0.5 indicates that we keep nominal of amount nonzero voxels across all
# maps, less the threshold means that more intense non-voxels will be survived.
from nilearn.regions import RegionExtractor
from nilearn import datasets
atlas = datasets.fetch_atlas_msdl()
# Loading atlas image stored in 'maps'
atlas_filename = atlas['maps']
extractor = RegionExtractor(components_img, threshold=1.0,
thresholding_strategy='ratio_n_voxels',
extractor='local_regions',
standardize=True, min_region_size=2000)
# Just call fit() to process for regions extraction
extractor.fit()
# Extracted regions are stored in regions_img_
regions_extracted_img = extractor.regions_img_
# Each region index is stored in index_
regions_index = extractor.index_
# Total number of regions extracted
n_regions_extracted = regions_extracted_img.shape[-1]
# Visualization of region extraction results
title = ('%d regions are extracted from %d components.'
'\nEach separate color of region indicates extracted region'
% (n_regions_extracted, 20))
plot_prob_atlas(regions_extracted_img, view_type='filled_contours',
title=title)
/usr/local/lib/python3.8/dist-packages/numpy/lib/npyio.py:2405: VisibleDeprecationWarning: Reading unicode strings without specifying the encoding argument is deprecated. Set the encoding, use None for the system default.
output = genfromtxt(fname, **kwargs)
/home/jt/.local/lib/python3.8/site-packages/nilearn/image/image.py:1054: FutureWarning: The parameter "sessions" will be removed in 0.9.0 release of Nilearn. Please use the parameter "runs" instead.
data = signal.clean(
/usr/local/lib/python3.8/dist-packages/numpy/ma/core.py:2825: UserWarning: Warning: converting a masked element to nan.
_data = np.array(data, dtype=dtype, copy=copy,
/home/jt/.local/lib/python3.8/site-packages/nilearn/plotting/displays.py:101: UserWarning: No contour levels were found within the data range.
im = getattr(ax, type)(data_2d.copy(),
/home/jt/.local/lib/python3.8/site-packages/nilearn/plotting/displays.py:101: UserWarning: linewidths is ignored by contourf
im = getattr(ax, type)(data_2d.copy(),
<nilearn.plotting.displays.OrthoSlicer at 0x7f9aae611820>
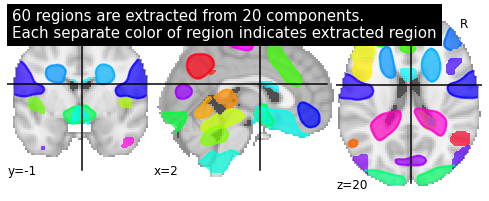
Timeseries extraction¶
Now we are ready to extract the different timeseries of the subjects.
# First we need to do subjects timeseries signals extraction and then estimating
# correlation matrices on those signals.
# To extract timeseries signals, we call transform() from RegionExtractor object
# onto each subject functional data stored in files_list.
# To estimate correlation matrices we import connectome utilities from nilearn
from nilearn.connectome import ConnectivityMeasure
correlations = []
# Initializing ConnectivityMeasure object with kind='correlation'
connectome_measure = ConnectivityMeasure(kind='correlation')
for filename in files_list:
# call transform from RegionExtractor object to extract timeseries signals
timeseries_each_subject = extractor.transform(filename)
# call fit_transform from ConnectivityMeasure object
correlation = connectome_measure.fit_transform([timeseries_each_subject])
# saving each subject correlation to correlations
correlations.append(correlation)
# Mean of all correlations
import numpy as np
mean_correlations = np.mean(correlations, axis=0).reshape(n_regions_extracted,
n_regions_extracted)
Atlas import¶
Importing the atlas for labeling the matrices later.
from nilearn import image
from nilearn import input_data
from nilearn import datasets
msdl_atlas_dataset = datasets.fetch_atlas_msdl()
# A "memory" to avoid recomputation
from joblib import Memory
mem = Memory('nilearn_cache')
masker = input_data.NiftiMapsMasker(
msdl_atlas_dataset.maps, resampling_target="maps", detrend=True,
high_variance_confounds=True, low_pass=None, high_pass=0.01,
t_r=2, standardize=True, memory='nilearn_cache', memory_level=1,
verbose=2)
masker.fit()
subject_time_series = []
func_filenames = files_list
for func_filename in (func_filenames):
print("Processing file %s" % func_filename)
region_ts = masker.transform(func_filename)
subject_time_series.append(region_ts)
/usr/local/lib/python3.8/dist-packages/numpy/lib/npyio.py:2405: VisibleDeprecationWarning: Reading unicode strings without specifying the encoding argument is deprecated. Set the encoding, use None for the system default.
output = genfromtxt(fname, **kwargs)
[NiftiMapsMasker.fit] loading regions from /home/jt/nilearn_data/msdl_atlas/MSDL_rois/msdl_rois.nii
Processing file /media/jt/Daten1/preproc/datasink/smooth/sub-52/task-restingstatewithclosedeyes/fwhm-8_swarsub-52_task-rest_bold.nii
/home/jt/.local/lib/python3.8/site-packages/nilearn/image/image.py:1054: FutureWarning: The parameter "sessions" will be removed in 0.9.0 release of Nilearn. Please use the parameter "runs" instead.
data = signal.clean(
[Memory]0.0s, 0.0min : Loading high_variance_confounds...
[Memory]0.1s, 0.0min : Loading filter_and_extract...
Processing file /media/jt/Daten1/preproc/datasink/smooth/sub-53/task-restingstatewithclosedeyes/fwhm-8_swarsub-53_task-rest_bold.nii
[Memory]0.1s, 0.0min : Loading high_variance_confounds...
________________________________________________________________________________
[Memory] Calling nilearn.input_data.base_masker.filter_and_extract...
filter_and_extract('/media/jt/Daten1/preproc/datasink/smooth/sub-53/task-restingstatewithclosedeyes/fwhm-8_swarsub-53_task-rest_bold.nii',
<nilearn.input_data.nifti_maps_masker._ExtractionFunctor object at 0x7f9aac7b0d60>,
{ 'allow_overlap': True,
'detrend': True,
'dtype': None,
'high_pass': 0.01,
'high_variance_confounds': True,
'low_pass': None,
'maps_img': '/home/jt/nilearn_data/msdl_atlas/MSDL_rois/msdl_rois.nii',
'mask_img': None,
'smoothing_fwhm': None,
'standardize': True,
'standardize_confounds': True,
't_r': 2,
'target_affine': array([[ 4., 0., 0., -78.],
[ 0., 4., 0., -111.],
[ 0., 0., 4., -51.],
[ 0., 0., 0., 1.]]),
'target_shape': (40, 48, 35)}, confounds=[ array([[0.08794 , ..., 0.056381],
...,
[0.126701, ..., 0.08743 ]])], sample_mask=None, dtype=None, memory=Memory(location=nilearn_cache/joblib), memory_level=1, verbose=2)
[NiftiMapsMasker.transform_single_imgs] Loading data from /media/jt/Daten1/preproc/datasink/smooth/sub-53/task-restingstatewithclosedeyes/fwhm-8_swarsub-53_task-rest_bold.nii
[NiftiMapsMasker.transform_single_imgs] Resampling images
[NiftiMapsMasker.transform_single_imgs] Extracting region signals
[NiftiMapsMasker.transform_single_imgs] Cleaning extracted signals
_______________________________________________filter_and_extract - 6.9s, 0.1min
Processing file /media/jt/Daten1/preproc/datasink/smooth/sub-54/task-restingstatewithclosedeyes/fwhm-8_swarsub-54_task-rest_bold.nii
[Memory]7.1s, 0.1min : Loading high_variance_confounds...
[Memory]7.2s, 0.1min : Loading filter_and_extract...
Processing file /media/jt/Daten1/preproc/datasink/smooth/sub-55/task-restingstatewithclosedeyes/fwhm-8_swarsub-55_task-rest_bold.nii
[Memory]7.2s, 0.1min : Loading high_variance_confounds...
[Memory]7.2s, 0.1min : Loading filter_and_extract...
Processing file /media/jt/Daten1/preproc/datasink/smooth/sub-56/task-restingstatewithclosedeyes/fwhm-8_swarsub-56_task-rest_bold.nii
[Memory]7.2s, 0.1min : Loading high_variance_confounds...
[Memory]7.3s, 0.1min : Loading filter_and_extract...
Processing file /media/jt/Daten1/preproc/datasink/smooth/sub-57/task-restingstatewithclosedeyes/fwhm-8_swarsub-57_task-rest_bold.nii
[Memory]7.3s, 0.1min : Loading high_variance_confounds...
[Memory]7.4s, 0.1min : Loading filter_and_extract...
Processing file /media/jt/Daten1/preproc/datasink/smooth/sub-58/task-restingstatewithclosedeyes/fwhm-8_swarsub-58_task-rest_bold.nii
[Memory]7.4s, 0.1min : Loading high_variance_confounds...
[Memory]7.5s, 0.1min : Loading filter_and_extract...
Processing file /media/jt/Daten1/preproc/datasink/smooth/sub-59/task-restingstatewithclosedeyes/fwhm-8_swarsub-59_task-rest_bold.nii
[Memory]7.5s, 0.1min : Loading high_variance_confounds...
[Memory]7.5s, 0.1min : Loading filter_and_extract...
Processing file /media/jt/Daten1/preproc/datasink/smooth/sub-60/task-restingstatewithclosedeyes/fwhm-8_swarsub-60_task-rest_bold.nii
[Memory]7.5s, 0.1min : Loading high_variance_confounds...
[Memory]7.6s, 0.1min : Loading filter_and_extract...
Processing file /media/jt/Daten1/preproc/datasink/smooth/sub-61/task-restingstatewithclosedeyes/fwhm-8_swarsub-61_task-rest_bold.nii
[Memory]7.6s, 0.1min : Loading high_variance_confounds...
[Memory]7.7s, 0.1min : Loading filter_and_extract...
Processing file /media/jt/Daten1/preproc/datasink/smooth/sub-62/task-restingstatewithclosedeyes/fwhm-8_swarsub-62_task-rest_bold.nii
[Memory]7.7s, 0.1min : Loading high_variance_confounds...
[Memory]7.8s, 0.1min : Loading filter_and_extract...
Processing file /media/jt/Daten1/preproc/datasink/smooth/sub-63/task-restingstatewithclosedeyes/fwhm-8_swarsub-63_task-rest_bold.nii
[Memory]7.8s, 0.1min : Loading high_variance_confounds...
[Memory]7.8s, 0.1min : Loading filter_and_extract...
Processing file /media/jt/Daten1/preproc/datasink/smooth/sub-64/task-restingstatewithclosedeyes/fwhm-8_swarsub-64_task-rest_bold.nii
[Memory]7.8s, 0.1min : Loading high_variance_confounds...
[Memory]7.9s, 0.1min : Loading filter_and_extract...
Processing file /media/jt/Daten1/preproc/datasink/smooth/sub-65/task-restingstatewithclosedeyes/fwhm-8_swarsub-65_task-rest_bold.nii
[Memory]7.9s, 0.1min : Loading high_variance_confounds...
[Memory]8.0s, 0.1min : Loading filter_and_extract...
Processing file /media/jt/Daten1/preproc/datasink/smooth/sub-66/task-restingstatewithclosedeyes/fwhm-8_swarsub-66_task-rest_bold.nii
[Memory]8.0s, 0.1min : Loading high_variance_confounds...
[Memory]8.1s, 0.1min : Loading filter_and_extract...
Processing file /media/jt/Daten1/preproc/datasink/smooth/sub-67/task-restingstatewithclosedeyes/fwhm-8_swarsub-67_task-rest_bold.nii
[Memory]8.1s, 0.1min : Loading high_variance_confounds...
[Memory]8.1s, 0.1min : Loading filter_and_extract...
Processing file /media/jt/Daten1/preproc/datasink/smooth/sub-68/task-restingstatewithclosedeyes/fwhm-8_swarsub-68_task-rest_bold.nii
[Memory]8.1s, 0.1min : Loading high_variance_confounds...
[Memory]8.2s, 0.1min : Loading filter_and_extract...
Processing file /media/jt/Daten1/preproc/datasink/smooth/sub-69/task-restingstatewithclosedeyes/fwhm-8_swarsub-69_task-rest_bold.nii
[Memory]8.2s, 0.1min : Loading high_variance_confounds...
[Memory]8.3s, 0.1min : Loading filter_and_extract...
Processing file /media/jt/Daten1/preproc/datasink/smooth/sub-70/task-restingstatewithclosedeyes/fwhm-8_swarsub-70_task-rest_bold.nii
[Memory]8.3s, 0.1min : Loading high_variance_confounds...
[Memory]8.3s, 0.1min : Loading filter_and_extract...
Processing file /media/jt/Daten1/preproc/datasink/smooth/sub-71/task-restingstatewithclosedeyes/fwhm-8_swarsub-71_task-rest_bold.nii
[Memory]8.3s, 0.1min : Loading high_variance_confounds...
[Memory]8.4s, 0.1min : Loading filter_and_extract...
Processing file /media/jt/Daten1/preproc/datasink/smooth/sub-72/task-restingstatewithclosedeyes/fwhm-8_swarsub-72_task-rest_bold.nii
[Memory]8.4s, 0.1min : Loading high_variance_confounds...
[Memory]8.5s, 0.1min : Loading filter_and_extract...
Correlation matrices and connectomes¶
We use two different ways to compute the precision and correlation, GraphicalLassoCV and GroupSparseCovarianceCV.
from nilearn.connectome import GroupSparseCovarianceCV
gsc = GroupSparseCovarianceCV(verbose=2)
gsc.fit(subject_time_series)
try:
from sklearn.covariance import GraphicalLassoCV
except ImportError:
# for Scitkit-Learn < v0.20.0
from sklearn.covariance import GraphLassoCV as GraphicalLassoCV
gl = GraphicalLassoCV(verbose=2)
gl.fit(np.concatenate(subject_time_series))
[Parallel(n_jobs=1)]: Using backend SequentialBackend with 1 concurrent workers.
[GroupSparseCovarianceCV.fit] Log-likelihood on test set is decreasing. Stopping at iteration 5
[GroupSparseCovarianceCV.fit] Log-likelihood on test set is decreasing. Stopping at iteration 0
[Parallel(n_jobs=1)]: Done 1 out of 1 | elapsed: 6.0s remaining: 0.0s
[GroupSparseCovarianceCV.fit] Log-likelihood on test set is decreasing. Stopping at iteration 2
[GroupSparseCovarianceCV.fit] Log-likelihood on test set is decreasing. Stopping at iteration 0
[GroupSparseCovarianceCV.fit] Log-likelihood on test set is decreasing. Stopping at iteration 5
[GroupSparseCovarianceCV.fit] Log-likelihood on test set is decreasing. Stopping at iteration 9
[GroupSparseCovarianceCV.fit] Log-likelihood on test set is decreasing. Stopping at iteration 0
[GroupSparseCovarianceCV.fit] Log-likelihood on test set is decreasing. Stopping at iteration 9
[GroupSparseCovarianceCV.fit] Log-likelihood on test set is decreasing. Stopping at iteration 0
[GroupSparseCovarianceCV.fit] Log-likelihood on test set is decreasing. Stopping at iteration 10
[GroupSparseCovarianceCV.fit] Log-likelihood on test set is decreasing. Stopping at iteration 0
[GroupSparseCovarianceCV.fit] [GroupSparseCovarianceCV] Done refinement 1 out of 4
[Parallel(n_jobs=1)]: Done 5 out of 5 | elapsed: 29.6s finished
[Parallel(n_jobs=1)]: Using backend SequentialBackend with 1 concurrent workers.
[GroupSparseCovarianceCV.fit] Log-likelihood on test set is decreasing. Stopping at iteration 0
[Parallel(n_jobs=1)]: Done 1 out of 1 | elapsed: 11.0s remaining: 0.0s
[GroupSparseCovarianceCV.fit] Log-likelihood on test set is decreasing. Stopping at iteration 8
[GroupSparseCovarianceCV.fit] Log-likelihood on test set is decreasing. Stopping at iteration 0
[GroupSparseCovarianceCV.fit] Log-likelihood on test set is decreasing. Stopping at iteration 8
[GroupSparseCovarianceCV.fit] Log-likelihood on test set is decreasing. Stopping at iteration 7
[GroupSparseCovarianceCV.fit] Log-likelihood on test set is decreasing. Stopping at iteration 0
[GroupSparseCovarianceCV.fit] Log-likelihood on test set is decreasing. Stopping at iteration 6
[GroupSparseCovarianceCV.fit] Log-likelihood on test set is decreasing. Stopping at iteration 8
[GroupSparseCovarianceCV.fit] Log-likelihood on test set is decreasing. Stopping at iteration 0
[GroupSparseCovarianceCV.fit] Log-likelihood on test set is decreasing. Stopping at iteration 7
[GroupSparseCovarianceCV.fit] Log-likelihood on test set is decreasing. Stopping at iteration 7
[GroupSparseCovarianceCV.fit] Log-likelihood on test set is decreasing. Stopping at iteration 0
[GroupSparseCovarianceCV.fit] [GroupSparseCovarianceCV] Done refinement 2 out of 4
[Parallel(n_jobs=1)]: Done 5 out of 5 | elapsed: 56.1s finished
[Parallel(n_jobs=1)]: Using backend SequentialBackend with 1 concurrent workers.
[GroupSparseCovarianceCV.fit] Log-likelihood on test set is decreasing. Stopping at iteration 4
[Parallel(n_jobs=1)]: Done 1 out of 1 | elapsed: 8.3s remaining: 0.0s
[GroupSparseCovarianceCV.fit] Log-likelihood on test set is decreasing. Stopping at iteration 10
[GroupSparseCovarianceCV.fit] Log-likelihood on test set is decreasing. Stopping at iteration 9
[GroupSparseCovarianceCV.fit] Log-likelihood on test set is decreasing. Stopping at iteration 6
[GroupSparseCovarianceCV.fit] Log-likelihood on test set is decreasing. Stopping at iteration 6
[GroupSparseCovarianceCV.fit] Log-likelihood on test set is decreasing. Stopping at iteration 8
[Parallel(n_jobs=1)]: Done 5 out of 5 | elapsed: 50.6s finished
[Parallel(n_jobs=1)]: Using backend SequentialBackend with 1 concurrent workers.
[GroupSparseCovarianceCV.fit] [GroupSparseCovarianceCV] Done refinement 3 out of 4
[GroupSparseCovarianceCV.fit] Log-likelihood on test set is decreasing. Stopping at iteration 6
[Parallel(n_jobs=1)]: Done 1 out of 1 | elapsed: 8.4s remaining: 0.0s
[GroupSparseCovarianceCV.fit] Log-likelihood on test set is decreasing. Stopping at iteration 13
[GroupSparseCovarianceCV.fit] Log-likelihood on test set is decreasing. Stopping at iteration 7
[GroupSparseCovarianceCV.fit] Log-likelihood on test set is decreasing. Stopping at iteration 8
[GroupSparseCovarianceCV.fit] Log-likelihood on test set is decreasing. Stopping at iteration 6
[GroupSparseCovarianceCV.fit] Log-likelihood on test set is decreasing. Stopping at iteration 9
[GroupSparseCovarianceCV.fit] Log-likelihood on test set is decreasing. Stopping at iteration 8
[GroupSparseCovarianceCV.fit] Log-likelihood on test set is decreasing. Stopping at iteration 8
[Parallel(n_jobs=1)]: Done 5 out of 5 | elapsed: 57.3s finished
[GroupSparseCovarianceCV.fit] [GroupSparseCovarianceCV] Done refinement 4 out of 4
[GroupSparseCovarianceCV.fit] Final optimization
[GroupSparseCovarianceCV.fit] tolerance reached at iteration number 23: 8.801e-04
[Parallel(n_jobs=1)]: Using backend SequentialBackend with 1 concurrent workers.
....[Parallel(n_jobs=1)]: Done 1 out of 1 | elapsed: 0.5s remaining: 0.0s
................[Parallel(n_jobs=1)]: Done 5 out of 5 | elapsed: 2.5s finished
[Parallel(n_jobs=1)]: Using backend SequentialBackend with 1 concurrent workers.
.
[GraphicalLassoCV] Done refinement 1 out of 4: 2s
...[Parallel(n_jobs=1)]: Done 1 out of 1 | elapsed: 0.3s remaining: 0.0s
................[Parallel(n_jobs=1)]: Done 5 out of 5 | elapsed: 2.6s finished
[Parallel(n_jobs=1)]: Using backend SequentialBackend with 1 concurrent workers.
[GraphicalLassoCV] Done refinement 2 out of 4: 5s
....[Parallel(n_jobs=1)]: Done 1 out of 1 | elapsed: 0.6s remaining: 0.0s
................[Parallel(n_jobs=1)]: Done 5 out of 5 | elapsed: 2.4s finished
[Parallel(n_jobs=1)]: Using backend SequentialBackend with 1 concurrent workers.
.
[GraphicalLassoCV] Done refinement 3 out of 4: 7s
...[Parallel(n_jobs=1)]: Done 1 out of 1 | elapsed: 0.5s remaining: 0.0s
...............
[GraphicalLassoCV] Done refinement 4 out of 4: 10s
[graphical_lasso] Iteration 0, cost 1.62e+02, dual gap 4.862e-01
[graphical_lasso] Iteration 1, cost 1.62e+02, dual gap -1.406e-02
[graphical_lasso] Iteration 2, cost 1.62e+02, dual gap -8.801e-04
[graphical_lasso] Iteration 3, cost 1.62e+02, dual gap -1.949e-04
[graphical_lasso] Iteration 4, cost 1.62e+02, dual gap 6.202e-05
.[Parallel(n_jobs=1)]: Done 5 out of 5 | elapsed: 2.6s finished
[Parallel(n_jobs=1)]: Using backend SequentialBackend with 1 concurrent workers.
[Parallel(n_jobs=1)]: Done 5 out of 5 | elapsed: 0.0s finished
GraphicalLassoCV(verbose=2)
Define a function to plot the matrices easier.
import numpy as np
from nilearn import plotting
def plot_matrices(cov, prec, title, labels):
"""Plot covariance and precision matrices, for a given processing. """
prec = prec.copy() # avoid side effects
# Put zeros on the diagonal, for graph clarity.
size = prec.shape[0]
prec[list(range(size)), list(range(size))] = 0
span = max(abs(prec.min()), abs(prec.max()))
# Display covariance matrix
plotting.plot_matrix(cov, cmap=plotting.cm.bwr,
vmin=-1, vmax=1, title="%s / covariance" % title,
labels=labels)
# Display precision matrix
plotting.plot_matrix(prec, cmap=plotting.cm.bwr,
vmin=-span, vmax=span, title="%s / precision" % title,
labels=labels)
Plotting the connectomes and matrices.
atlas_img = msdl_atlas_dataset.maps
atlas_region_coords = plotting.find_probabilistic_atlas_cut_coords(atlas_img)
labels = msdl_atlas_dataset.labels
plotting.plot_connectome(gl.covariance_,
atlas_region_coords, edge_threshold='90%',
title="Covariance",
display_mode="lzr")
plotting.plot_connectome(-gl.precision_, atlas_region_coords,
edge_threshold='90%',
title="Sparse inverse covariance (GraphicalLasso)",
display_mode="lzr",
edge_vmax=.5, edge_vmin=-.5)
plot_matrices(gl.covariance_, gl.precision_, "GraphicalLasso", labels)
title = "GroupSparseCovariance"
plotting.plot_connectome(-gsc.precisions_[..., 0],
atlas_region_coords, edge_threshold='90%',
title=title,
display_mode="lzr",
edge_vmax=.5, edge_vmin=-.5)
plot_matrices(gsc.covariances_[..., 0],
gsc.precisions_[..., 0], title, labels)
import numpy as np
import matplotlib.pyplot as plt
plotting.show()
Saving the matrices.
from nilearn import plotting
#1st matrix
cov=gsc.covariances_[..., 0]
title='GroupSparseCovariance'
display=plotting.plot_matrix(cov, cmap=plotting.cm.bwr,
vmin=-1, vmax=1, title="%s / covariance" % title,
labels=labels)
display.figure.savefig('GSC_covariance_healthy.png', dpi=300)
plotting.show()
# 2nd matrix
cov=gl.covariance_
title='GraphicLassoCV'
# Display covariance matrix
display=plotting.plot_matrix(cov, cmap=plotting.cm.bwr,
vmin=-1, vmax=1, title="%s / covariance" % title,
labels=labels)
display.figure.savefig('GL_covariances_healthy.png', dpi=300)
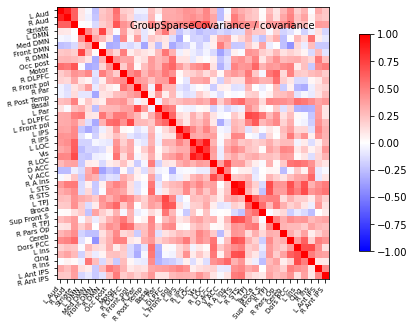
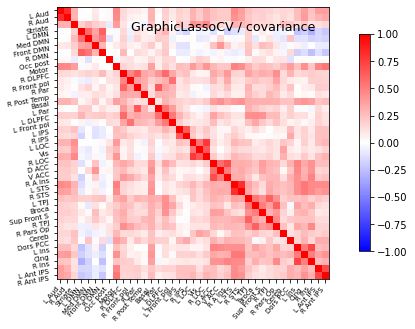
Saving the precision matrix.
prec = gsc.precisions_[..., 0]
# Put zeros on the diagonal, for graph clarity.
size = prec.shape[0]
prec[list(range(size)), list(range(size))] = 0
span = max(abs(prec.min()), abs(prec.max()))
title='GSC'
# Display precision matrix
display=plotting.plot_matrix(prec, cmap=plotting.cm.bwr,
vmin=-span, vmax=span, title="%s / precision" % title,
labels=labels)
display.figure.savefig('Precision_healthy.png', dpi=300)
plotting.show()
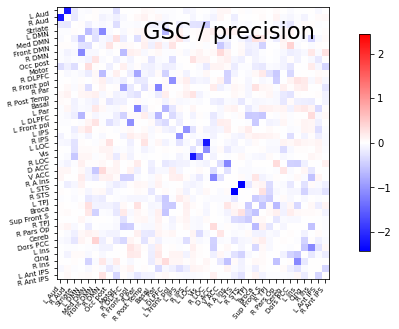
Matrices are nice to see but we look also for the exactly figures and saving the as .csv file.
import pandas as pd
import opendyxl
df=pd.DataFrame(data=gl.covariance_, index=labels, columns=labels)
df.to_csv('GL_covariances_healthy.csv')
df.to_excel('GL_covariances_healthyx.xlsx')
df
L Aud | R Aud | Striate | L DMN | Med DMN | Front DMN | R DMN | Occ post | Motor | R DLPFC | ... | Sup Front S | R TPJ | R Pars Op | Cereb | Dors PCC | L Ins | Cing | R Ins | L Ant IPS | R Ant IPS | |
---|---|---|---|---|---|---|---|---|---|---|---|---|---|---|---|---|---|---|---|---|---|
L Aud | 1.000000 | 0.821322 | 0.302834 | -0.007970 | -0.000290 | 0.083248 | -0.008446 | 0.025825 | 0.471992 | 0.153481 | ... | 0.187710 | 0.164365 | 0.139000 | 0.160901 | 0.141476 | 0.254763 | 0.382023 | 0.234387 | 0.380239 | 0.381568 |
R Aud | 0.821322 | 1.000000 | 0.296288 | 0.003880 | 0.008565 | 0.096501 | -0.007617 | 0.029980 | 0.455368 | 0.135232 | ... | 0.191201 | 0.145730 | 0.106199 | 0.160628 | 0.123911 | 0.253044 | 0.394793 | 0.226349 | 0.300582 | 0.342406 |
Striate | 0.302834 | 0.296288 | 1.000000 | 0.186124 | 0.271864 | 0.192337 | 0.155576 | -0.072835 | 0.385552 | 0.114155 | ... | 0.162618 | 0.149518 | 0.104844 | 0.240618 | 0.216173 | 0.155662 | 0.223019 | 0.112553 | 0.121608 | 0.163799 |
L DMN | -0.007970 | 0.003880 | 0.186124 | 1.000000 | 0.568449 | 0.357647 | 0.621197 | 0.017152 | -0.020522 | 0.143032 | ... | 0.078178 | 0.029761 | -0.057754 | -0.046867 | -0.015133 | -0.237673 | -0.187451 | -0.311885 | -0.162219 | -0.216600 |
Med DMN | -0.000290 | 0.008565 | 0.271864 | 0.568449 | 1.000000 | 0.406085 | 0.537214 | -0.114467 | 0.030808 | 0.179529 | ... | 0.026600 | 0.107054 | -0.064841 | -0.096755 | 0.233878 | -0.121988 | -0.111553 | -0.172688 | -0.149471 | -0.173618 |
Front DMN | 0.083248 | 0.096501 | 0.192337 | 0.357647 | 0.406085 | 1.000000 | 0.288223 | 0.014671 | 0.085228 | 0.154653 | ... | 0.253364 | 0.210990 | 0.101415 | -0.039521 | -0.019831 | 0.047874 | -0.068672 | -0.006849 | -0.070474 | -0.077322 |
R DMN | -0.008446 | -0.007617 | 0.155576 | 0.621197 | 0.537214 | 0.288223 | 1.000000 | -0.000791 | -0.003168 | 0.270195 | ... | 0.082336 | 0.123463 | 0.050905 | -0.035837 | 0.143137 | -0.182998 | -0.125727 | -0.242219 | -0.121337 | -0.216231 |
Occ post | 0.025825 | 0.029980 | -0.072835 | 0.017152 | -0.114467 | 0.014671 | -0.000791 | 1.000000 | -0.001135 | 0.097417 | ... | 0.131882 | 0.036918 | 0.104544 | 0.112441 | -0.121793 | 0.000832 | 0.008614 | -0.001215 | 0.007786 | 0.012463 |
Motor | 0.471992 | 0.455368 | 0.385552 | -0.020522 | 0.030808 | 0.085228 | -0.003168 | -0.001135 | 1.000000 | 0.165353 | ... | 0.252036 | 0.238690 | 0.193177 | 0.278199 | 0.385122 | 0.246856 | 0.434211 | 0.220535 | 0.470407 | 0.543659 |
R DLPFC | 0.153481 | 0.135232 | 0.114155 | 0.143032 | 0.179529 | 0.154653 | 0.270195 | 0.097417 | 0.165353 | 1.000000 | ... | 0.321722 | 0.294848 | 0.438051 | 0.132872 | 0.294629 | 0.150487 | 0.171525 | 0.199701 | 0.175174 | 0.160887 |
R Front pol | 0.090199 | 0.061096 | -0.020731 | -0.054577 | -0.077080 | 0.063931 | 0.004791 | 0.123451 | 0.131287 | 0.475963 | ... | 0.194443 | 0.198761 | 0.356307 | 0.151952 | 0.085606 | 0.062017 | 0.054231 | 0.179745 | 0.182504 | 0.169799 |
R Par | 0.082842 | 0.045551 | 0.015581 | 0.116605 | 0.168084 | 0.040501 | 0.139942 | 0.041772 | 0.100957 | 0.598103 | ... | 0.144742 | 0.194803 | 0.263445 | 0.123862 | 0.233774 | 0.012741 | 0.064376 | 0.085485 | 0.198065 | 0.191991 |
R Post Temp | 0.049299 | 0.028759 | -0.042399 | 0.074442 | 0.067802 | 0.126845 | 0.110943 | 0.055722 | 0.120440 | 0.345676 | ... | 0.136595 | 0.277342 | 0.218744 | 0.083171 | 0.150238 | 0.012798 | 0.032666 | 0.020682 | 0.126716 | 0.091872 |
Basal | 0.293397 | 0.298721 | 0.237604 | 0.034606 | 0.087721 | 0.209381 | 0.041621 | 0.150458 | 0.374672 | 0.299768 | ... | 0.306169 | 0.309486 | 0.303961 | 0.240406 | 0.234051 | 0.378826 | 0.409864 | 0.368342 | 0.223995 | 0.264720 |
L Par | 0.038724 | 0.006267 | 0.018585 | 0.198444 | 0.196092 | 0.046650 | 0.126490 | 0.068537 | 0.065448 | 0.407329 | ... | 0.177270 | 0.146219 | 0.168545 | 0.072936 | 0.180735 | -0.017631 | -0.013019 | -0.037227 | 0.154378 | 0.063706 |
L DLPFC | 0.189240 | 0.178889 | 0.167735 | 0.291603 | 0.184528 | 0.202449 | 0.211260 | 0.158801 | 0.201661 | 0.579410 | ... | 0.424281 | 0.256344 | 0.269194 | 0.102848 | 0.182041 | 0.126304 | 0.125625 | 0.037122 | 0.176544 | 0.114093 |
L Front pol | 0.101292 | 0.079799 | -0.005493 | 0.042976 | -0.057301 | 0.052793 | 0.006960 | 0.144922 | 0.117738 | 0.350634 | ... | 0.212497 | 0.145577 | 0.231343 | 0.127833 | 0.043466 | 0.083730 | 0.023498 | 0.076699 | 0.171604 | 0.122877 |
L IPS | 0.185593 | 0.165473 | 0.242066 | -0.095714 | 0.009421 | -0.103951 | -0.075683 | -0.003154 | 0.250275 | 0.207079 | ... | 0.070490 | 0.007272 | 0.066221 | 0.068836 | 0.260098 | 0.107705 | 0.173213 | 0.082931 | 0.242332 | 0.239697 |
R IPS | 0.154596 | 0.134784 | 0.157066 | -0.166227 | -0.014219 | -0.110309 | -0.073261 | -0.021868 | 0.226967 | 0.247797 | ... | 0.005393 | -0.011959 | 0.043072 | 0.029259 | 0.336249 | 0.095358 | 0.169415 | 0.106054 | 0.239140 | 0.243863 |
L LOC | 0.301305 | 0.290510 | 0.434894 | 0.004414 | -0.003290 | 0.010256 | 0.024905 | 0.094088 | 0.391818 | 0.091549 | ... | 0.112125 | 0.157845 | 0.104516 | 0.226057 | 0.168417 | 0.045463 | 0.186614 | 0.078860 | 0.249903 | 0.284169 |
Vis | 0.203971 | 0.202653 | 0.383973 | -0.042707 | -0.081309 | -0.008658 | -0.042281 | 0.189283 | 0.278535 | 0.040542 | ... | 0.093351 | 0.134375 | 0.110457 | 0.361208 | 0.088744 | 0.052644 | 0.120770 | 0.092525 | 0.118581 | 0.176818 |
R LOC | 0.313199 | 0.311419 | 0.464972 | -0.031381 | -0.065442 | 0.031449 | -0.029851 | 0.149916 | 0.410608 | 0.050728 | ... | 0.148316 | 0.169889 | 0.103624 | 0.305301 | 0.126406 | 0.104072 | 0.205753 | 0.110457 | 0.238209 | 0.293147 |
D ACC | 0.232764 | 0.231001 | 0.164774 | -0.124237 | 0.020185 | 0.228909 | -0.042850 | 0.018620 | 0.275641 | 0.339441 | ... | 0.378599 | 0.295964 | 0.269199 | 0.150493 | 0.310226 | 0.484166 | 0.343619 | 0.419070 | 0.254878 | 0.256491 |
V ACC | 0.202841 | 0.207131 | 0.163315 | 0.004689 | 0.150581 | 0.408956 | 0.023530 | 0.046572 | 0.218681 | 0.269586 | ... | 0.360700 | 0.280829 | 0.241310 | 0.038629 | 0.221479 | 0.466850 | 0.303506 | 0.415477 | 0.158720 | 0.182960 |
R A Ins | 0.196484 | 0.196715 | 0.110820 | -0.174658 | 0.019088 | 0.191395 | -0.054506 | -0.009445 | 0.221985 | 0.401941 | ... | 0.231585 | 0.282738 | 0.280365 | 0.125656 | 0.306673 | 0.429995 | 0.316121 | 0.484290 | 0.210877 | 0.248302 |
L STS | 0.468286 | 0.431932 | 0.294314 | -0.129197 | -0.025204 | 0.052367 | -0.075085 | -0.022441 | 0.492357 | 0.164106 | ... | 0.149366 | 0.323007 | 0.280883 | 0.244855 | 0.287381 | 0.436098 | 0.528017 | 0.455170 | 0.447582 | 0.459581 |
R STS | 0.375290 | 0.360383 | 0.272816 | -0.079989 | 0.022888 | 0.086924 | -0.044104 | 0.000069 | 0.448073 | 0.158939 | ... | 0.118219 | 0.339665 | 0.264614 | 0.230148 | 0.279079 | 0.440639 | 0.518736 | 0.455719 | 0.385502 | 0.416224 |
L TPJ | 0.243757 | 0.218514 | 0.234190 | 0.181047 | 0.182090 | 0.223194 | 0.239269 | 0.059679 | 0.311787 | 0.360459 | ... | 0.429981 | 0.610054 | 0.436324 | 0.184282 | 0.242136 | 0.229173 | 0.261252 | 0.187385 | 0.233892 | 0.192395 |
Broca | 0.193543 | 0.171426 | 0.150992 | 0.016112 | -0.091393 | 0.118263 | 0.020755 | 0.135943 | 0.203117 | 0.277786 | ... | 0.506996 | 0.374532 | 0.616148 | 0.242585 | -0.025230 | 0.360803 | 0.263134 | 0.317845 | 0.152654 | 0.124832 |
Sup Front S | 0.187710 | 0.191201 | 0.162618 | 0.078178 | 0.026600 | 0.253364 | 0.082336 | 0.131882 | 0.252036 | 0.321722 | ... | 1.000000 | 0.308623 | 0.398679 | 0.165966 | 0.059869 | 0.277703 | 0.303780 | 0.225180 | 0.079876 | 0.109288 |
R TPJ | 0.164365 | 0.145730 | 0.149518 | 0.029761 | 0.107054 | 0.210990 | 0.123463 | 0.036918 | 0.238690 | 0.294848 | ... | 0.308623 | 1.000000 | 0.448582 | 0.143203 | 0.212642 | 0.228464 | 0.231618 | 0.241349 | 0.146508 | 0.136421 |
R Pars Op | 0.139000 | 0.106199 | 0.104844 | -0.057754 | -0.064841 | 0.101415 | 0.050905 | 0.104544 | 0.193177 | 0.438051 | ... | 0.398679 | 0.448582 | 1.000000 | 0.248490 | 0.096225 | 0.341226 | 0.282980 | 0.468211 | 0.168444 | 0.172094 |
Cereb | 0.160901 | 0.160628 | 0.240618 | -0.046867 | -0.096755 | -0.039521 | -0.035837 | 0.112441 | 0.278199 | 0.132872 | ... | 0.165966 | 0.143203 | 0.248490 | 1.000000 | 0.118694 | 0.168586 | 0.262298 | 0.195770 | 0.169950 | 0.207874 |
Dors PCC | 0.141476 | 0.123911 | 0.216173 | -0.015133 | 0.233878 | -0.019831 | 0.143137 | -0.121793 | 0.385122 | 0.294629 | ... | 0.059869 | 0.212642 | 0.096225 | 0.118694 | 1.000000 | 0.172550 | 0.304543 | 0.154578 | 0.317983 | 0.317387 |
L Ins | 0.254763 | 0.253044 | 0.155662 | -0.237673 | -0.121988 | 0.047874 | -0.182998 | 0.000832 | 0.246856 | 0.150487 | ... | 0.277703 | 0.228464 | 0.341226 | 0.168586 | 0.172550 | 1.000000 | 0.509702 | 0.720206 | 0.250896 | 0.281506 |
Cing | 0.382023 | 0.394793 | 0.223019 | -0.187451 | -0.111553 | -0.068672 | -0.125727 | 0.008614 | 0.434211 | 0.171525 | ... | 0.303780 | 0.231618 | 0.282980 | 0.262298 | 0.304543 | 0.509702 | 1.000000 | 0.501177 | 0.353148 | 0.427102 |
R Ins | 0.234387 | 0.226349 | 0.112553 | -0.311885 | -0.172688 | -0.006849 | -0.242219 | -0.001215 | 0.220535 | 0.199701 | ... | 0.225180 | 0.241349 | 0.468211 | 0.195770 | 0.154578 | 0.720206 | 0.501177 | 1.000000 | 0.256307 | 0.310489 |
L Ant IPS | 0.380239 | 0.300582 | 0.121608 | -0.162219 | -0.149471 | -0.070474 | -0.121337 | 0.007786 | 0.470407 | 0.175174 | ... | 0.079876 | 0.146508 | 0.168444 | 0.169950 | 0.317983 | 0.250896 | 0.353148 | 0.256307 | 1.000000 | 0.713751 |
R Ant IPS | 0.381568 | 0.342406 | 0.163799 | -0.216600 | -0.173618 | -0.077322 | -0.216231 | 0.012463 | 0.543659 | 0.160887 | ... | 0.109288 | 0.136421 | 0.172094 | 0.207874 | 0.317387 | 0.281506 | 0.427102 | 0.310489 | 0.713751 | 1.000000 |
39 rows × 39 columns
import pandas as pd
df=pd.DataFrame(data=gsc.covariances_[..., 0], index=labels, columns=labels)
df.to_csv('GSC_covariances_healthy.csv')
df.to_excel('GSC_covariances_healthyx.xlsx')
df
L Aud | R Aud | Striate | L DMN | Med DMN | Front DMN | R DMN | Occ post | Motor | R DLPFC | ... | Sup Front S | R TPJ | R Pars Op | Cereb | Dors PCC | L Ins | Cing | R Ins | L Ant IPS | R Ant IPS | |
---|---|---|---|---|---|---|---|---|---|---|---|---|---|---|---|---|---|---|---|---|---|
L Aud | 1.000000 | 0.863683 | 0.617724 | 0.098714 | -0.038518 | -0.249383 | 0.214160 | 0.280954 | 0.562076 | 0.197000 | ... | 0.010756 | 0.251799 | 0.108332 | 0.286633 | 0.452958 | 0.182039 | 0.291300 | -0.015151 | 0.333270 | 0.226463 |
R Aud | 0.863683 | 1.000000 | 0.617721 | 0.089870 | -0.062214 | -0.221921 | 0.230728 | 0.284349 | 0.523373 | 0.265652 | ... | 0.017269 | 0.311556 | 0.123411 | 0.350935 | 0.476658 | 0.235669 | 0.355265 | 0.052710 | 0.256362 | 0.213105 |
Striate | 0.617724 | 0.617721 | 1.000000 | -0.002543 | -0.015340 | -0.147667 | 0.191735 | 0.260023 | 0.661112 | 0.418348 | ... | 0.131641 | 0.385253 | 0.394537 | 0.440898 | 0.573878 | 0.219765 | 0.272360 | 0.019144 | 0.386343 | 0.370689 |
L DMN | 0.098714 | 0.089870 | -0.002543 | 1.000000 | 0.468413 | 0.242627 | 0.732315 | 0.208571 | -0.020530 | 0.377757 | ... | 0.201256 | -0.025664 | 0.191462 | -0.166859 | -0.113443 | -0.016284 | -0.178655 | -0.054978 | 0.120895 | -0.157023 |
Med DMN | -0.038518 | -0.062214 | -0.015340 | 0.468413 | 1.000000 | 0.358505 | 0.267446 | 0.105625 | -0.332911 | 0.462978 | ... | -0.231487 | 0.172158 | 0.318773 | -0.243839 | 0.008547 | 0.266959 | 0.138646 | 0.533224 | 0.072065 | -0.091328 |
Front DMN | -0.249383 | -0.221921 | -0.147667 | 0.242627 | 0.358505 | 1.000000 | 0.020555 | -0.327625 | -0.302263 | -0.115094 | ... | 0.356667 | -0.075448 | -0.002901 | -0.245528 | -0.415967 | 0.152029 | -0.070452 | 0.255722 | -0.209726 | -0.209270 |
R DMN | 0.214160 | 0.230728 | 0.191735 | 0.732315 | 0.267446 | 0.020555 | 1.000000 | 0.309723 | 0.159567 | 0.506717 | ... | 0.189947 | 0.133763 | 0.273897 | 0.110393 | 0.172848 | 0.120622 | 0.041138 | -0.146768 | 0.266023 | -0.117087 |
Occ post | 0.280954 | 0.284349 | 0.260023 | 0.208571 | 0.105625 | -0.327625 | 0.309723 | 1.000000 | 0.436726 | 0.349386 | ... | 0.095442 | 0.297827 | 0.433008 | 0.267675 | 0.300594 | 0.315028 | 0.236679 | 0.158979 | 0.531184 | 0.246319 |
Motor | 0.562076 | 0.523373 | 0.661112 | -0.020530 | -0.332911 | -0.302263 | 0.159567 | 0.436726 | 1.000000 | 0.199175 | ... | 0.301348 | 0.402235 | 0.381977 | 0.456285 | 0.498775 | 0.073883 | 0.243153 | -0.207019 | 0.451179 | 0.509541 |
R DLPFC | 0.197000 | 0.265652 | 0.418348 | 0.377757 | 0.462978 | -0.115094 | 0.506717 | 0.349386 | 0.199175 | 1.000000 | ... | -0.033459 | 0.529589 | 0.616645 | 0.146605 | 0.491149 | 0.217553 | 0.190786 | 0.265542 | 0.289921 | 0.199656 |
R Front pol | 0.175172 | 0.151742 | 0.337171 | 0.096099 | 0.042222 | -0.154827 | 0.070121 | 0.360025 | 0.414448 | 0.447553 | ... | 0.291114 | 0.305566 | 0.555590 | 0.190847 | 0.098767 | -0.142668 | -0.143536 | 0.035429 | 0.086932 | 0.265731 |
R Par | -0.056265 | -0.130900 | 0.109065 | 0.197550 | 0.385632 | -0.084284 | 0.160306 | 0.073085 | 0.028622 | 0.540249 | ... | -0.073152 | 0.005799 | 0.263497 | -0.198660 | 0.058353 | -0.286765 | -0.326332 | -0.066347 | 0.133251 | 0.176060 |
R Post Temp | -0.058607 | -0.112293 | 0.132831 | -0.094292 | -0.097608 | -0.346255 | 0.108240 | 0.129448 | 0.255634 | 0.287204 | ... | -0.018564 | 0.061989 | 0.238827 | 0.253080 | 0.319255 | -0.112975 | -0.037645 | -0.083054 | 0.109733 | 0.258758 |
Basal | 0.246614 | 0.278579 | 0.497192 | 0.044168 | 0.212060 | 0.107701 | 0.062677 | 0.397596 | 0.385045 | 0.428605 | ... | 0.147499 | 0.474688 | 0.450959 | 0.437951 | 0.396407 | 0.558655 | 0.523047 | 0.453100 | 0.349112 | 0.401747 |
L Par | 0.114251 | -0.022683 | 0.253416 | 0.309464 | 0.150099 | -0.255156 | 0.265104 | 0.276264 | 0.286058 | 0.363528 | ... | 0.060928 | -0.006891 | 0.208697 | -0.123654 | 0.171116 | -0.295453 | -0.319893 | -0.301753 | 0.372632 | 0.104013 |
L DLPFC | 0.327765 | 0.329731 | 0.506915 | 0.533326 | 0.105751 | 0.092034 | 0.534682 | 0.343345 | 0.517286 | 0.520164 | ... | 0.533014 | 0.269854 | 0.430546 | 0.115135 | 0.254498 | 0.027725 | -0.025712 | -0.219035 | 0.386861 | 0.108401 |
L Front pol | 0.321243 | 0.329730 | 0.402161 | 0.206709 | -0.129653 | -0.332488 | 0.303244 | 0.565817 | 0.594370 | 0.428648 | ... | 0.276463 | 0.365627 | 0.468301 | 0.170708 | 0.316795 | -0.019660 | -0.051831 | -0.195211 | 0.427902 | 0.266165 |
L IPS | 0.358925 | 0.346315 | 0.457581 | -0.220455 | -0.334492 | -0.212700 | -0.058052 | 0.117064 | 0.384478 | 0.023705 | ... | -0.016487 | 0.099262 | 0.025999 | 0.259064 | 0.382313 | 0.154415 | 0.162005 | -0.118903 | 0.483901 | 0.311552 |
R IPS | 0.414261 | 0.406437 | 0.461358 | -0.112557 | -0.006351 | -0.174636 | 0.058637 | 0.115169 | 0.245524 | 0.153396 | ... | -0.203918 | 0.156086 | 0.005029 | 0.224335 | 0.521559 | 0.257601 | 0.422030 | 0.061399 | 0.420052 | 0.296065 |
L LOC | 0.405826 | 0.441864 | 0.612385 | -0.165534 | -0.166337 | -0.038346 | -0.067808 | -0.087135 | 0.410428 | 0.186923 | ... | 0.217479 | 0.316033 | 0.199553 | 0.259015 | 0.258130 | -0.046830 | 0.075561 | -0.126432 | 0.066074 | 0.281090 |
Vis | 0.339165 | 0.386046 | 0.626448 | -0.256204 | -0.158590 | -0.175875 | -0.127423 | 0.047275 | 0.460224 | 0.253088 | ... | 0.016937 | 0.366403 | 0.279568 | 0.473632 | 0.450453 | 0.086427 | 0.213910 | 0.047861 | 0.126561 | 0.413887 |
R LOC | 0.475830 | 0.464593 | 0.702892 | -0.198359 | -0.224618 | -0.069656 | -0.041324 | -0.008453 | 0.544032 | 0.137534 | ... | 0.236872 | 0.254056 | 0.214555 | 0.380842 | 0.366753 | 0.022631 | 0.096719 | -0.129461 | 0.161979 | 0.302948 |
D ACC | 0.268615 | 0.345578 | 0.364229 | -0.215911 | 0.074334 | 0.057308 | -0.143043 | 0.067667 | 0.111652 | 0.237225 | ... | -0.020335 | 0.299027 | 0.020192 | 0.208084 | 0.536850 | 0.567260 | 0.520747 | 0.427602 | 0.181426 | 0.106946 |
V ACC | -0.259859 | -0.261332 | -0.066578 | -0.140895 | 0.321070 | 0.504820 | -0.366593 | -0.226942 | -0.297528 | 0.024360 | ... | 0.000777 | 0.039841 | 0.033541 | -0.261632 | -0.029843 | 0.218249 | 0.050504 | 0.481297 | -0.079157 | -0.030099 |
R A Ins | -0.027444 | 0.049896 | 0.223767 | -0.199324 | 0.353980 | 0.294928 | -0.200007 | -0.115217 | -0.133970 | 0.322153 | ... | 0.015894 | 0.285604 | 0.183685 | 0.107181 | 0.251272 | 0.348722 | 0.363348 | 0.590687 | -0.093693 | 0.108526 |
L STS | 0.401666 | 0.511015 | 0.518347 | -0.147824 | 0.059715 | -0.213563 | 0.061774 | 0.380344 | 0.481922 | 0.404639 | ... | -0.142831 | 0.702452 | 0.420981 | 0.409243 | 0.680993 | 0.450022 | 0.675374 | 0.297009 | 0.432700 | 0.511402 |
R STS | 0.318138 | 0.370139 | 0.450342 | -0.136210 | 0.154265 | -0.206673 | -0.015915 | 0.424507 | 0.406091 | 0.337203 | ... | -0.234192 | 0.668367 | 0.384620 | 0.387711 | 0.637560 | 0.502269 | 0.701730 | 0.410388 | 0.472935 | 0.541172 |
L TPJ | 0.263684 | 0.249174 | 0.414783 | 0.322783 | 0.282775 | 0.204456 | 0.421714 | 0.309278 | 0.414136 | 0.529733 | ... | 0.461936 | 0.495612 | 0.495691 | 0.136983 | 0.298302 | 0.189329 | 0.192795 | 0.085847 | 0.333150 | 0.195162 |
Broca | 0.203966 | 0.213345 | 0.435676 | 0.215721 | -0.137514 | 0.074629 | 0.384610 | 0.483480 | 0.601410 | 0.217681 | ... | 0.565183 | 0.265933 | 0.537582 | 0.309475 | 0.173274 | 0.242596 | 0.094663 | -0.067953 | 0.397080 | 0.121494 |
Sup Front S | 0.010756 | 0.017269 | 0.131641 | 0.201256 | -0.231487 | 0.356667 | 0.189947 | 0.095442 | 0.301348 | -0.033459 | ... | 1.000000 | -0.018379 | 0.092047 | 0.155046 | -0.192770 | -0.084871 | -0.148297 | -0.252014 | 0.004419 | 0.092058 |
R TPJ | 0.251799 | 0.311556 | 0.385253 | -0.025664 | 0.172158 | -0.075448 | 0.133763 | 0.297827 | 0.402235 | 0.529589 | ... | -0.018379 | 1.000000 | 0.491947 | 0.313546 | 0.451619 | 0.393147 | 0.409340 | 0.273159 | 0.323639 | 0.356127 |
R Pars Op | 0.108332 | 0.123411 | 0.394537 | 0.191462 | 0.318773 | -0.002901 | 0.273897 | 0.433008 | 0.381977 | 0.616645 | ... | 0.092047 | 0.491947 | 1.000000 | 0.294258 | 0.272495 | 0.265882 | 0.200437 | 0.366367 | 0.283687 | 0.256529 |
Cereb | 0.286633 | 0.350935 | 0.440898 | -0.166859 | -0.243839 | -0.245528 | 0.110393 | 0.267675 | 0.456285 | 0.146605 | ... | 0.155046 | 0.313546 | 0.294258 | 1.000000 | 0.316352 | 0.201712 | 0.365100 | 0.065940 | 0.182917 | 0.303525 |
Dors PCC | 0.452958 | 0.476658 | 0.573878 | -0.113443 | 0.008547 | -0.415967 | 0.172848 | 0.300594 | 0.498775 | 0.491149 | ... | -0.192770 | 0.451619 | 0.272495 | 0.316352 | 1.000000 | 0.383100 | 0.622743 | 0.178025 | 0.495922 | 0.418616 |
L Ins | 0.182039 | 0.235669 | 0.219765 | -0.016284 | 0.266959 | 0.152029 | 0.120622 | 0.315028 | 0.073883 | 0.217553 | ... | -0.084871 | 0.393147 | 0.265882 | 0.201712 | 0.383100 | 1.000000 | 0.689485 | 0.698238 | 0.428314 | 0.118037 |
Cing | 0.291300 | 0.355265 | 0.272360 | -0.178655 | 0.138646 | -0.070452 | 0.041138 | 0.236679 | 0.243153 | 0.190786 | ... | -0.148297 | 0.409340 | 0.200437 | 0.365100 | 0.622743 | 0.689485 | 1.000000 | 0.505247 | 0.383370 | 0.366619 |
R Ins | -0.015151 | 0.052710 | 0.019144 | -0.054978 | 0.533224 | 0.255722 | -0.146768 | 0.158979 | -0.207019 | 0.265542 | ... | -0.252014 | 0.273159 | 0.366367 | 0.065940 | 0.178025 | 0.698238 | 0.505247 | 1.000000 | 0.098824 | 0.087081 |
L Ant IPS | 0.333270 | 0.256362 | 0.386343 | 0.120895 | 0.072065 | -0.209726 | 0.266023 | 0.531184 | 0.451179 | 0.289921 | ... | 0.004419 | 0.323639 | 0.283687 | 0.182917 | 0.495922 | 0.428314 | 0.383370 | 0.098824 | 1.000000 | 0.455064 |
R Ant IPS | 0.226463 | 0.213105 | 0.370689 | -0.157023 | -0.091328 | -0.209270 | -0.117087 | 0.246319 | 0.509541 | 0.199656 | ... | 0.092058 | 0.356127 | 0.256529 | 0.303525 | 0.418616 | 0.118037 | 0.366619 | 0.087081 | 0.455064 | 1.000000 |
39 rows × 39 columns
from PIL import Image
import urllib.request
URL = 'https://st.depositphotos.com/1431107/1631/i/950/depositphotos_16317151-stock-photo-well-done-illustration.jpg'
with urllib.request.urlopen(URL) as url:
with open('temp.jpg', 'wb') as f:
f.write(url.read())
img = Image.open('temp.jpg')
img.show()